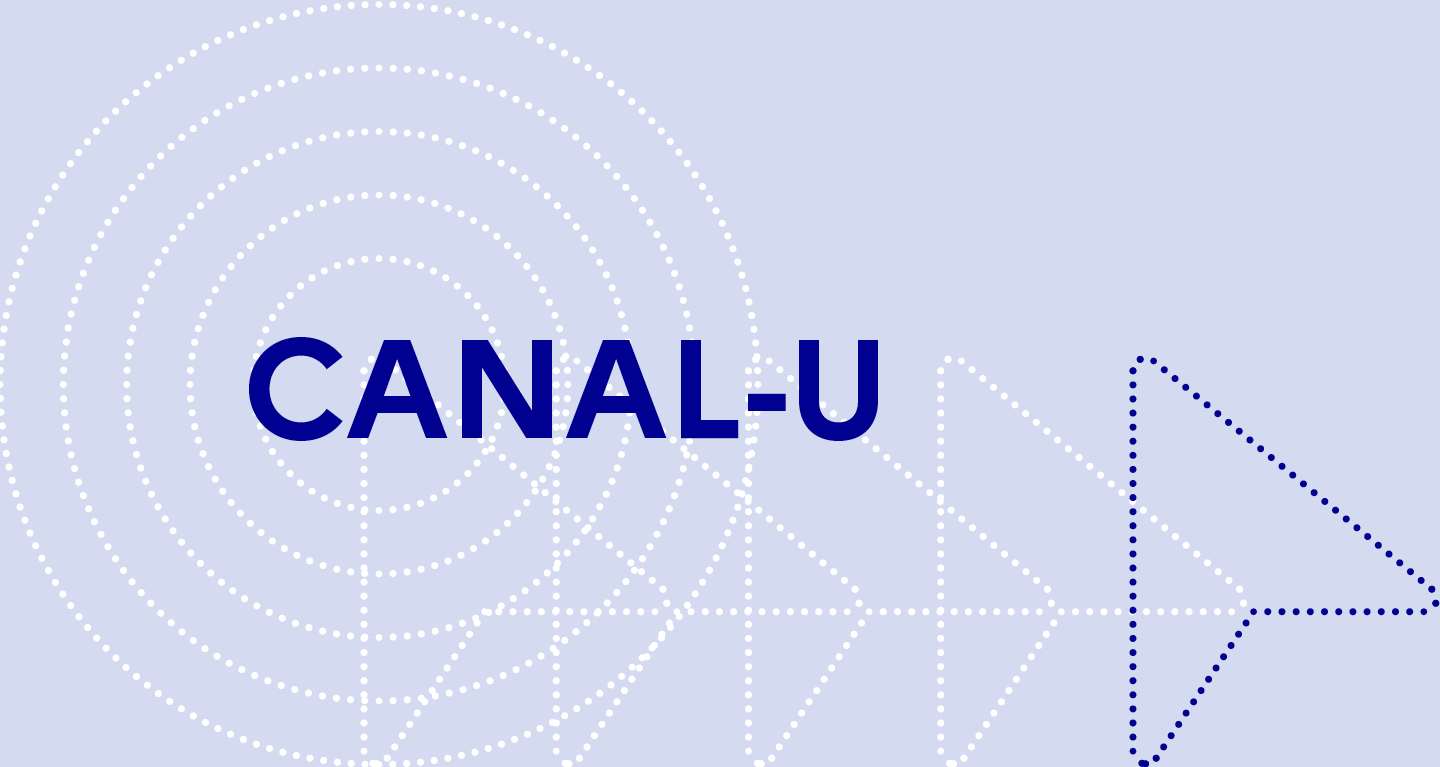
Sommaire
Action recognition from video: some recent results
Date de création :
01.12.2011Auteur(s) :
Cordelia SCHMIDPrésentation
Informations pratiques
Droits réservés à l'éditeur et aux auteurs.
Description de la ressource
Résumé
While recognition in still images has received a lot of attention over the past years, recognition in videos is just emerging. In this talk I will present some recent results. Bags of features have demonstrated good performance for action recognition in videos. We briefly review the underlying principles and introduce trajectory-based video features, which have shown to outperform the state of the art. These features are obtained by dense point sampling in each frame and tracking them based on displacement information from a dense optical flow field. Trajectory descriptors are obtained from motion boundary histograms, which are robust to camera motion. We then show how to integrate temporal structure into a bag-of-features model based on so-called actom sequences. We localize actions based on sequences of atomic actions, i.e., represent the temporal structure by sequences of histograms of actom-anchored visual features. This representation is flexible, sparse and discriminative. The resulting model is shown to significantly improve performance over existing methods for temporal action localization. Finally, we show how to move towards more structured representations by explicitly modeling human-object interactions. We learn how to represent human actions as interactions between persons and objects. We localize in space and track over time both the object and the person, and represent an action as the trajectory of the object with respect to the person position, i.e., our human-object interaction features capture the relative trajectory of the object with respect to the human. This is shown to improve over existing methods for action localization.
"Domaine(s)" et indice(s) Dewey
- Vision par ordinateur (006.37)
Domaine(s)
- Informatique
- Vision, perception
Intervenants, édition et diffusion
Intervenants
Édition
- Région PACA
- INRIA (Institut national de recherche en informatique et automatique)
Diffusion
Document(s) annexe(s)
Fiche technique
- LOMv1.0
- LOMFRv1.0
- Voir la fiche XML